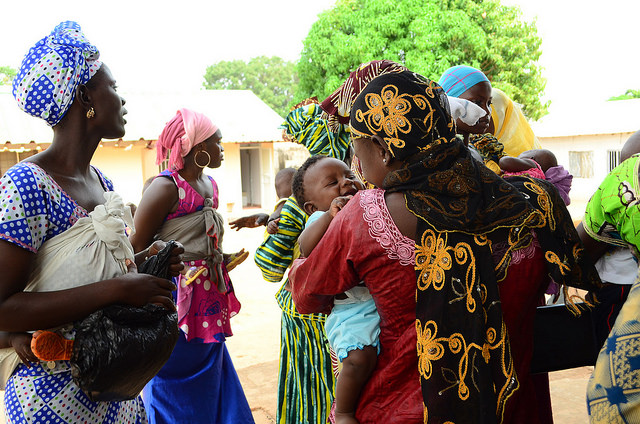
Photo credit: NAY_8628, Nayyar Photography, Flickr 2014, CC-BY 2.0.
Malaria affects half of the world’s population and is a huge burden to societies. Despite our best efforts, hundreds of millions of people are infected each year and close to one million die, primarily children under the age of five. Malaria infection is known to have a genetic component and some well-studied genetic changes causing sickle cell disease and other blood disorders have been linked to malaria resistance.
With this in mind, we set out to do what others have done with many human diseases – to perform a genome-wide association study to detect genetic variants that increase a person’s risk of being infected. In this type of study, we look simultaneously at hundreds of thousands of known genetic variants in children with severe malaria, and in healthy individuals from the same countries. If any of these known genetic variants are located close enough to a disease-causing variant, we can see an association with the disease… at least in theory.
In practice though, things didn’t quite work out as planned.
Our first challenge was purely technical. Because most DNA samples in our collection had come from young children, the samples were extremely precious and we had amplified them to make the amount of DNA we got last longer. It turned out that the process of amplifying the DNA made the DNA gloopy and difficult to measure. Our early studies had wildly variable success because the amount of DNA that went into genotyping, the process of identifying underlying variants, changed from one week to the next. After a few false starts, we managed to optimise DNA handling and since then the genotyping has worked well.
We also discovered that amplified DNA gave noisier genetic signals than genomic DNA, and available genotyping software failed to identify many variants. To overcome this, we developed new software – Illuminus – that could handle noisy data.
Our main challenges, however, were entirely due to long and complex ancestry of African people. We had overestimated the level of correlation between known variants and disease-causing variants, and underestimated how different the combinations of known genetic variants would be between countries in Africa.
Normally we would have overcome the low level of correlation by using public human sequencing data to essentially guess – or impute – unknown genetic variants and produce a very dense map of genetic variation. We tried this with our Gambian sample collection by using sequencing data from Nigeria but by doing this we lost association signal in the sickle cell disease variant, which should have shown a very strong association with malaria resistance!
So we returned to the drawing board to recruit more individuals and to test ever more thorough genotyping. Several iterations later, we finally have a sample collection that is big enough to detect association reliably, and a genotyping chip that has very high resolution to overcome the challenges caused by low levels of correlation between disease-causing mutations and known genetic variants. With this sample collection, we have been able to confirm that we can see association to the known malaria resistance variants, and we are following some new leads in a bigger sample collection to verify them.
We still have the challenge of guessing – or imputing –unknown genetic variants. The imputation of variants depends on the correlation between known variants and disease-causing variants as well as the similarity in the combinations of observed genotypes, or variants, between our sample collection and available sequencing data. Optimally we would impute genotypes, or variants, using sequencing data from people from the same countries – and preferably the same ethnic groups – that we have genotyped. This is exactly what we are doing right now and we will make the sequencing data public to help others studying disease associations in African populations.
Katja Kivinen joined the MalariaGEN Consortium and Sanger Institute Malaria Programme in 2007. She is involved in all stages of human malaria projects.
Reference
Band et al. Imputation-Based Meta-Analysis of Severe Malaria in Three African Populations. PLoS Genet. 2013 May;9(5):e1003509. doi: 10.1371/journal.pgen.1003509. Epub 2013 May 23.